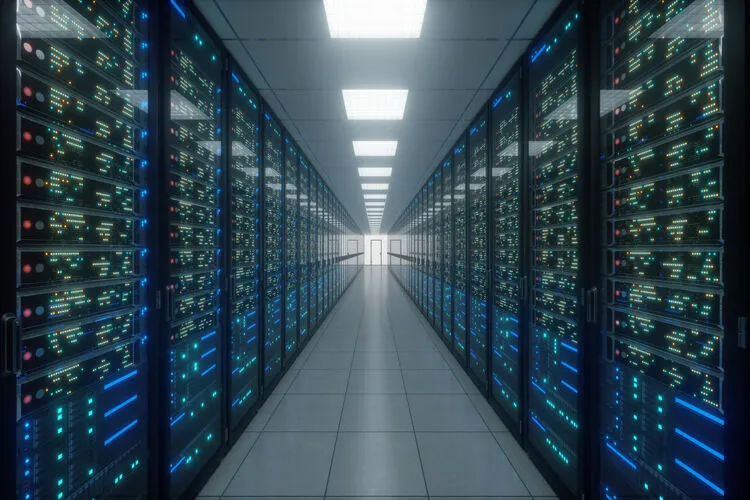
A simple search using AI uses ten times as much energy as Google. Here’s how AI is a quiet contributor to our planet’s pollution
By
More than ever, information is at our fingertips. It has never been easier to ask a question, whether that’s to an AI chatbot or a large language model such as ChatGPT. But all of this readily-accessed material comes at a cost, not only to the wallets of those creating these machines, but to our planet’s environment.
Google, Meta and Microsoft have reported increased greenhouse gas emissions since 2020, with constantly operating AI data systems found in data centres largely to blame. Microsoft alone reported a 40 per cent uptick in its emissions between 2020 and 2023, from the equivalent of 12.2 million tonnes of CO2 to 17.1 million tonnes.
Google’s emissions have rocketed by 50 per cent from 2019 to 2023, with its latest environmental report stating the difficulty of planned emission reductions ‘due to increasing energy demands from the greater intensity of AI compute.’
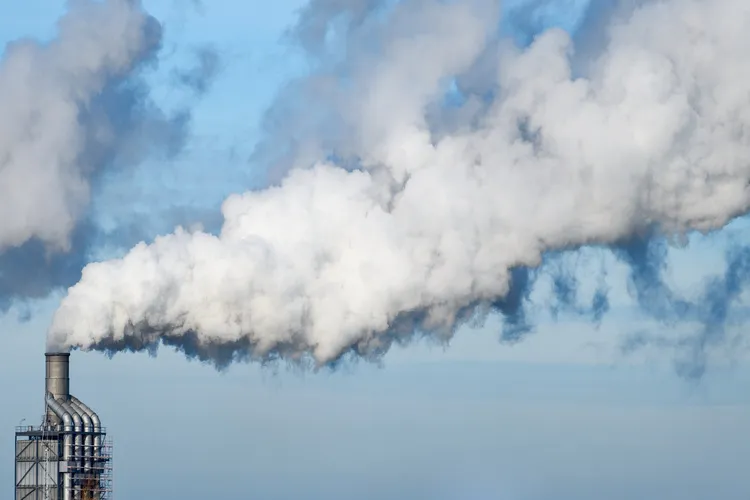
Let’s be clear: companies are under no illusions of the strain that AI is putting on the planet.
It’s worth noting that AI is not all doom and gloom: it’s ability to detect patterns has been used to help the environment in many different ways. The tech is useful for identifying weather patterns long before humans could. It can help tackle storms before they hit, icebergs before they calve, analyse air pollution and track soil moisture content to ensure an adequate water supply. Breakthroughs in green energy sources like nuclear fusion are also happening with AI.
Yet with computer power given to AI doubling every 100 days, we are tipping the scales in which the processes used to help our planet are no longer solely beneficial but will increasingly pollute it.
Is AI pollution inevitable?
By 2030, it is projected that data centre emissions will globally accumulate to 2.5 billion metric tonnes of CO2 equivalent. To put that into perspective, this is a figure equivalent to the emissions released by 642,613 coal power plants in an entire year. Insidious as it is, these emissions will continue to pollute the planet whether we realise it or not.
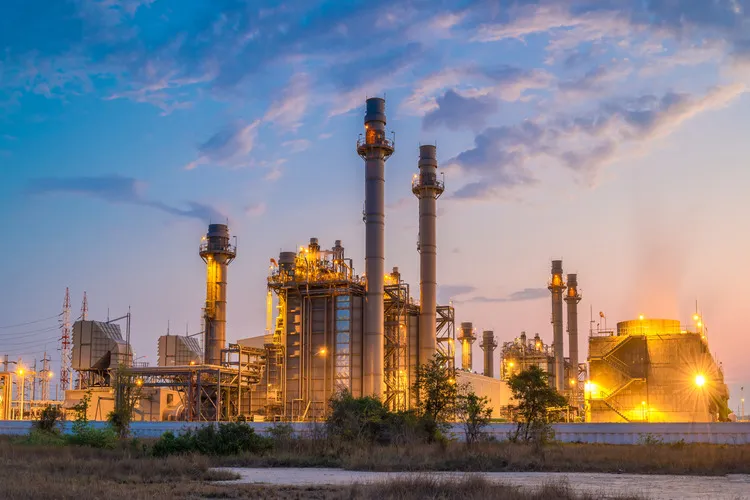
Data centres also use unfathomable quantities of water in two key ways. The first is by drawing electricity from power plants that use vast amounts of water to cool themselves. The second is within the data centres themselves, which get hot from so much electricity in them that they utilise cooling systems.
In 2021 – and this was before ChatGPT and the larger commercial AI boom took off – Google’s US data centres consumed an estimated 12.7 billion litres of fresh water. Training Microsoft’s GPT-3 AI program for just two weeks in US data centres consumed the same amount of water – 700,000 litres – as in the manufacturing process of 370 BMW cars or 320 Tesla electric vehicles. If the program was trained in Microsoft’s less efficient Asia data centres, this figure would be tripled.
Companies are beginning to tackle the impact that AI has on the planet. Plans to reduce the strain on water include projects such as improving community water conservation and designing watershed landscapes that are durable and resilient.
AI can also be trained at any time in the day, so switching to cooler times when less water is lost due to evaporation can help.
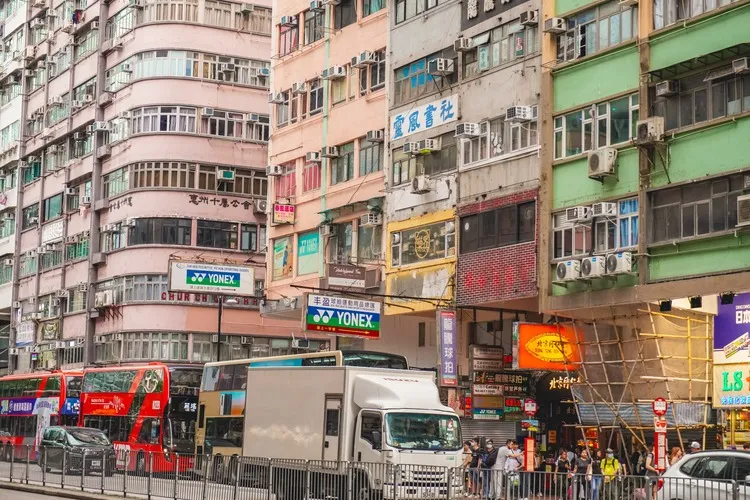
Data centres located near big cities can also end up competing with people for resources, particularly in heatwaves or droughts. In these situations, air conditioning is vital to ensure optimal public health. But with AI and people both wanting the same stuff, it could quickly lead down a slippery slope of blackouts and electricity grid instability. Using shared data centres rather than individual private infrastructure could help lessen the demand on energy resources, as well as scheduling training to occur during times of lower energy demand such as in the evening, as well as in cooler months.
Quantum computing – which doesn’t result in larger energy outputs as demand increases, like traditional computing – is also being considered as a long-term strategy to more AI into more sustainable territory. Large language models can also become more compact, and efficient using quantum computing without the need for more energy to update them.
Like most new innovations, AI brings both its benefits and added baggage. Time will tell whether more strategies will be devised to negate the heavy strain that AI places on energy consumption, but one thing is certain: with it firmly rising in popularity across a variety of sectors, the AI industry itself will not be slowing down.